Synergetics' 6 Pillars of AI
Successful AI Adoption & Projects
The application of Artificial Intelligence (AI) methods, technologies, and solutions represents a fundamental shift in how people interact with information. AI augments human experience, automates repeatable processes, and improves decision making with Machine Learning (ML), Natural Language Processing (NLP), and Robotic Process Automation (RPA). However, responsible adoption of AI must be measured by accepted success criteria.
Therefore, our test for AI readiness begins with our “6 Pillars of AI,” which ensure that the finished product provides:
- the right solutions for its users, and
- enduring value to the organization as a whole.
The 6 Pillars establish our attitude and approach when executing AI transformations for our customers.
We apply our pillars to every requirement, phase, recommendation, and action across the project lifecycle, leveraging them as a “value check” at each decision point.
According to McKinsey research, only 8% of companies use practices that enable effective adoption of AI. A common misconception is that AI is “plug and play.” Unfortunately, to make a valuable contribution, an organization needs:
- Data infrastructure
- Data expertise (talent)
- AI software tools (technology)
- Model development
While these four things are needed for successful AI use, broad AI adoption actually requires a change in a company’s mindset, culture, and strategy. In other words, leaders must know how to lead their organization in a significant transformation effort. We’ve outlined how leaders can succeed in such efforts through Kotter’s 8 Stages of Transformation.
AI will be most effective when an organization has centered all of its functions around using it. Project-based AI has its place and can be of value, but the more that a company shifts from seeing AI as a tool, to seeing it as a broad methodology, the more the promises of AI will be realized.
Therefore, in every AI project we use these 6 Pillars of AI to ensure that the solutions developed and the transformations made address broad organizational priorities (not merely isolated issues) and bring lasting value to the organization.
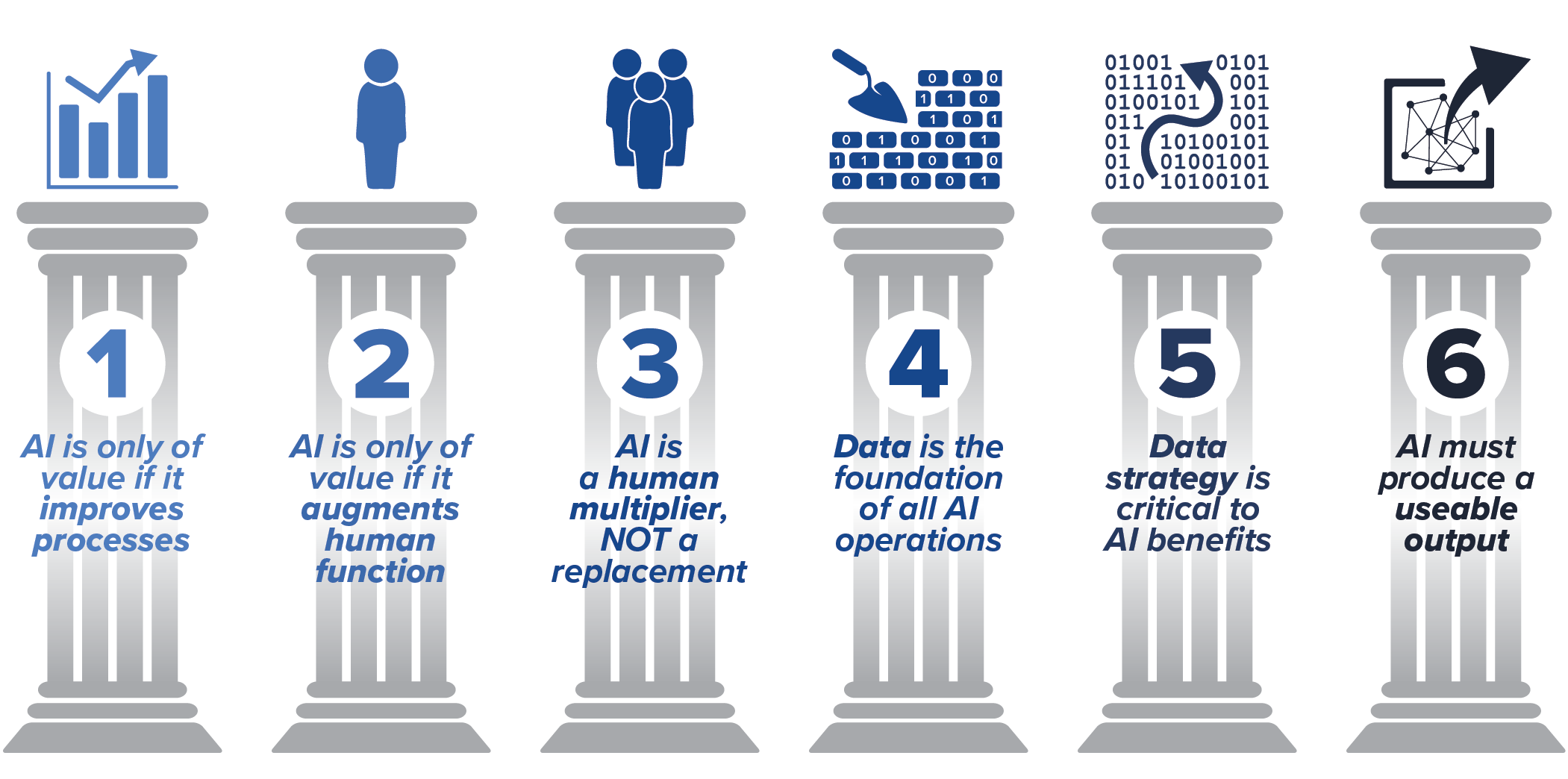
1. AI is only of value if it improves something
Currently, AI is a hot topic and a hot buy for Government agencies. It’s exciting, being talked about, seen as forward-thinking, and generally looks bright and shiny. This causes many organizations to get into trouble, because deep analysis isn’t made on how AI will bring broad, long-lasting value to the organization.
The first question we guide organizations to ask is this:
- What do you want to accomplish, and how do you imagine AI can help you reach that goal?
The second question organizations should ask is:
- Based on that goal, are the costs of implementing AI acceptable? Is the business impact worth the cost to the business?
The cost of AI is so much more than its sticker price; truly adopting AI in order to realize its full potential requires transforming an organization’s culture, vision, and strategy. Such broad transformation is not easy nor cheap, and thus needs to be taken into consideration when developing an AI strategy or planning an AI acquisition.
The aim of this pillar is to help an organization determine if AI is the best approach to their problem, given the desired outcome and the required investment.
2. AI is only of value if it augments human function
Though AI is supposed to make jobs easier and more efficient, an organization should carefully evaluate exactly how the proposed solution will do this. A valuable AI solution should decrease human dependencies.
In other words, if it takes your people longer to work with the AI solution, rather than to do the process “manually,” then the AI is not actually decreasing human dependency, and is therefore not doing what it should fundamentally do.
If your AI solution is failing here, before concluding that the AI solution itself is the problem, your organization should carefully evaluate the solution, including asking the following questions:
- How accurate is our data?
- How efficient is our data infrastructure?
- Are we using the right AI model?
- Is our team adopting and implementing the solution as intended?
This evaluation might uncover that your solution is valuable and will decrease human dependencies, if only your data were better, your infrastructure was efficient, or your team had fully adopted it as intended.
Ultimately, the process of using AI needs to be easier than the old way of doing things.
3. AI is a human multiplier, not a replacement
While AI that has the intelligence to operate independent of human input is theoretically possible, the vast majority of companies that could effectively use AI today will use it in such a manner that still depends on people to guide its use and make decisions.
Don’t imagine that an AI solution will be able to replace a person or a team in your organization; instead, an effective solution should turn your people into “super humans” – enabling them to process, for example, twice as much inputs as before.
AI should remove the repetitive subsets of a person’s job, enabling them to be more efficient, productive, and able to focus on high value tasks.
4. Data is the foundation of all AI operations
It hardly needs to be said that for a machine to learn, it has to have data from which to learn – the more, the better. An organization’s AI solution is only as good as the quantity and quality of the data its built upon.
To store the necessary data, such organizations must have large computational power, access to data science expertise, and data sets on which to train models.
In other words, from the beginning leaders should develop an infrastructure strategy that enables AI success.
5. Data strategy is critical to AI benefits
Data infrastructure alone is not enough for AI to be effective, much less to produce desired benefits for an organization.
Data strategy overall involves creating processes to collect records (particularly results and outcomes), which in turn produce data. Data is the required input for ML, and predictive/explanatory models (i.e., the desired outcome of AI) is dependent on effective ML.
Once data has been gathered, it’s vital for the data strategy to outline how that data can be verified, cleaned, and structured so that the data is accurate and usable.
The principle “garbage in, garbage out” succinctly describes the importance of a well-formed data strategy and its place as one of our pillars of AI.
6. AI must produce a usable output
Finally, the proposed (or developed!) AI solution must lead to a result that brings value – to the specific project in which it is implemented, and ultimately to the organization as a whole.
If, from the start, the effectiveness and scope of a proposed AI solution will be siloed or limited by an organization’s structure or culture, the value of that solution should be questioned. This doesn’t mean there isn’t value in small projects with quick turnaround and limited results – only that a successful AI output or even model is not the same as AI supporting a business process.
As the AI is used, your teams should be asking and validating the following two questions:
- Did the AI model produce a usable output?
- Is that output valuable for the business?
The Value of These Pillars
Together, these 6 Pillars of AI enable us and the organizations we work with to ensure that:
- AI is the right methodology for the problems faced
- The AI solutions developed are an effective approach to those problems
- The output from AI will ultimately lead to long-term business value for the organization
WANT TO KNOW MORE ABOUT THIS PRODUCT?
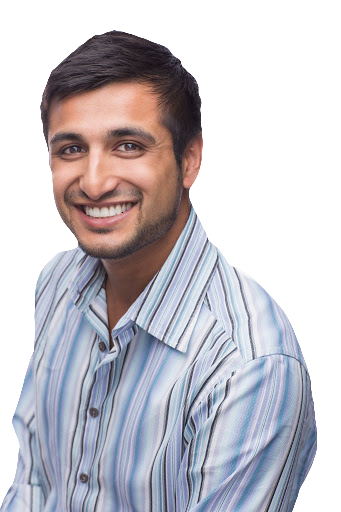
Kabir Mehta, COO